The Hidden Emissions in the Self-driving Vehicle Revolution
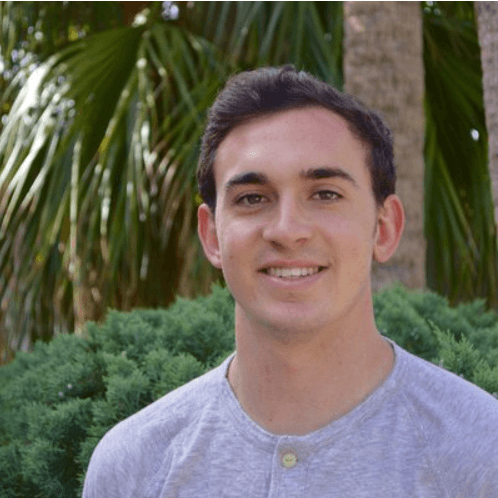
Zero car accidents. That is the promise of self-driving (or autonomous) vehicles. No more falling asleep at the wheel, being distracted by a phone call, or driving under the influence causing crashes. Indeed, the promised future of self-driving vehicles whirring us from one activity to the next has been anticipated for decades, and that future seems more apparent with every implementation of them in a factory warehouse, on another bus route, or with a new Tesla update. However, as exciting as safer and more convenient travel sounds, the environmental impact of this transportation option has remained in the background of the conversation. Though lives saved by reducing car accidents is an imperative, we must also consider the loss of life induced by climate change and an increasingly unlivable world. In this research, we explored the neglected environmental impacts of self-driving vehicle operation, and—in the environmental context—their future appears less bright.
While transportation already contributes around 27% of all greenhouse gas emissions in the United States, self-driving vehicles are poised to increase this due to the enormous data management required for their operation. The data management process starts with a connection between the vehicle and its surrounding environment: the roads, buildings, other vehicles, pedestrians, etc. This requires the vehicles to continuously use sensors to “perceive” the world around them (collect data), then use high-functioning computers to “act” on the interpretation of their data-driven perception (process data). For improved training of the vehicles or networked coordination between vehicles, the data must be transferred, stored, and secured. Each of these stages requires an enormous amount of energy.
During the last few years, awareness of the environmental challenges with data management has increased due to the popularity of cryptocurrencies, generative AI, and massive media companies like Google, Facebook, and Amazon. The Cloud’s carbon footprint has surpassed the airline industry, and the energy for data centers alone required one percent of all global energy in 2023, according to the International Energy Administration. This is because computational processes require energy to process data, as well as considerations like cooling the hardware. That required energy must come from sources such as coal, petroleum, and solar power, and whichever source of energy it is, it likely has a carbon footprint associated with it.
If the energy requirements and environmental impact are significantly large for these existing technologies, what might we expect for self-driving vehicles? To answer that question, we considered a United States-sized fleet of passenger vehicles converted to fully autonomous capabilities—no human intervention required. Using 15 editable variables, ranging from market penetration to the energy required by each individual LiDAR sensor on various vehicle models, we established estimates for the carbon footprint of this emerging technology’s data management needs.
Our findings paint a cautious picture of the full-scale adoption of self-driving vehicles. For instance, a full transition of passenger vehicles in the United States to self-driving vehicles would have data management-related emissions equivalent to one-fourth of Japan’s total country emissions. In the context of a renewable energy transition, transitioning only 40% of passenger vehicles in the U.S. would have data management energy requirements equal to 11% of the nation’s current solar capacity. Devoting such a sizable chunk of energy generation capabilities to managing data puts it in direct competition with arguably more basic energy needs like heating and cooling, food production, and medical devices. Thus, the adoption of self-driving vehicles would only intensify an already significant need for renewable energy production.
Though our estimates are promising, they err on the conservative side. We neglected the possibility that more data is collected, shared, and stored for purposes beyond vehicle operation such as policing, real estate, infrastructure maintenance, or marketing. McKinsey & Co. have identified such outcomes as likely, which would drive the energy requirements and environmental impact of the data management further.
Our research also has broader implications for the automation revolution occurring outside the transportation sector. Automation is being applied to agriculture, manufacturing, and medicine to name a few. Such an expansion of data requirements and its management will further exacerbate the environmental footprint associated with computational energy requirements. Though automation may make life more convenient—and even make the operation of some systems more efficient—the overall data management process places environmental pressures in conflict with our collective goals. Thus, as we consider ways to reduce vehicle accidents and make life convenient, we may look to other innovative ways without such large environmental impacts: walkable communities, bikeable infrastructure, and public transportation systems.
About the Author:
Kendrick Hardaway is part of the Interdisciplinary Ecological Sciences and Engineering Program at Purdue University. He holds a bachelor’s degree in biological engineering from the University of Arkansas, and he is a candidate for a master’s in industrial engineering and a doctorate in environmental engineering at Purdue. He is advised by Drs. Hua Cai and Roshanak Nateghi. In his research, he models interactions between social, ecological, and technical systems to quantify the ecological impacts of emerging technologies and to build resilient infrastructure systems.
April 08, 2024